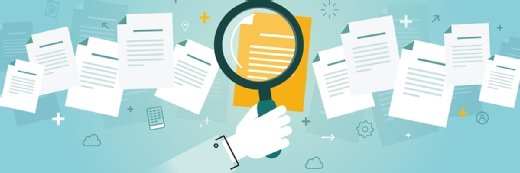
Data management key to GenAI success
Deloitte survey shows business and IT leaders are optimistic, but academic researchers warn of AI training time bomb
Business and IT executives interviewed for a recent Deloitte survey are wary of the costs associated with generative artificial intelligence (GenAI) projects, and the clock is ticking for organisations to create significant and sustained value through their GenAI initiatives.
According to Deloitte, cost will increasingly become a key factor in decision-making over GenAI. The survey of 2,770 business and IT leaders reported that only 16% of organisations said they produce regular reports for the chief financial officer about the value being created with the technology.
However, Deloitte said that as GenAI becomes an integral part of how business gets done, its initiatives will increasingly be measured against traditional financial metrics as organisations start to demand more tangible and measurable results from their GenAI investments.
In the State of generative AI in the enterprise report accompanying the poll, Deloitte predicted that businesses will adopt a comprehensive set of financial and non-financial measures to present a complete picture of the value created from investments in GenAI initiatives.
“In the future, we may see new metrics emerge that reflect its unique characteristics and capabilities,” the report’s authors wrote. “For example, there could be a metric that quantifies the performance of human workers and GenAI systems (together vs. separately) on creative and innovation-related tasks.”
Deloitte urged business and IT leaders to work out how to measure and communicate the technology’s value, which it said is critical for setting expectations and maintaining interest, support and investment from the C-suite and boardroom. It quotes a senior director and head of a GenAI accelerator in the pharmaceutical industry who believes the performance of large language models (LLMs) still needs to be improved along with data readiness. “Data is going to be problem forever,” they said. “Deep generative AI understanding, as well. There’s not enough people who understand and can drive transformation.”
Deloitte said the value from GenAI initiatives will increasingly come from organisations making use of differentiated data in new ways, such as for fine-tuning LLMs, building an LLM from scratch or developing enterprise AI applications. “For generative AI to deliver the kind of impact executives expect, companies will likely need to increase their comfort with using their proprietary data, which may be subject to existing and emerging regulations,” the report’s authors said.
Access to public data for training
There are many cases where organisations are able to enhance LLMs trained on internet data with internal data to customise public AI-based systems to their particular business requirements. However, research has found that using public LLM data as the source to train another LLM may lead to inaccuracies.
In an article published in Nature last month, researchers Ilia Shumailov, Zakhar Shumaylov, Yiren Zhao, Nicolas Papernot, Ross Anderson and Yarin Ga claimed there was a “first mover advantage” when it comes to training AI models.
“In our work, we demonstrate that training on samples from another generative model can induce a distribution shift, which – over time – causes model collapse,” they said.
The researchers recommended that the AI model developers ensured access to the original data source is preserved and that further data not generated by LLMs remained available over time. They also warned about the difficulty in identifying data created by LLMs on the internet.
“The need to distinguish data generated by LLMs from other data raises questions about the provenance of content that is crawled from the internet,” they noted.
The researchers also predicted it could become increasingly difficult to train newer versions of LLMs without access to data crawled from the internet before the mass adoption of the technology or direct access to data generated by humans at scale.
A possible solution suggested by the researchers is that different parties involved in LLM creation and deployment could share the information needed to determine the original source of the data.
Read more about LLMs
- AI- or human-generated? To test their reliability, six popular generative AI detectors were asked to judge three pieces of content. The one they got wrong may surprise you.
- As scepticism creeps more and more into the generative AI discussion, I’ve been trying to gain a clearer view of where real value lies, particularly for tools used directly by end users.